Example of the sklearn interface usage over the bosk pipeline#
Scikit-learn is a standart instrument that uses every data scientist, so probably you would like to get an adapter between bosk
pipeline and a scikit-learn model. We’ve developed such classes for classifier and regression models.
Let’s make a classification bosk
model.
[1]:
from bosk.executor.sklearn_interface import BoskPipelineClassifier
from bosk.painter.graphviz import GraphvizPainter
from bosk.pipeline.builder.functional import FunctionalPipelineBuilder
from sklearn.datasets import load_iris
from sklearn.model_selection import train_test_split
from sklearn.metrics import ConfusionMatrixDisplay
from IPython.display import Image
[2]:
n_estimators = 20
b = FunctionalPipelineBuilder()
X, y = b.Input()(), b.TargetInput()()
rf_1 = b.RFC(n_estimators=n_estimators)(X=X, y=y)
et_1 = b.ETC(n_estimators=n_estimators)(X=X, y=y)
concat_1 = b.Concat(['X', 'rf_1', 'et_1'])(X=X, rf_1=rf_1, et_1=et_1)
rf_2 = b.RFC(n_estimators=n_estimators)(X=concat_1, y=y)
et_2 = b.ETC(n_estimators=n_estimators)(X=concat_1, y=y)
stack = b.Stack(['rf_2', 'et_2'], axis=1)(rf_2=rf_2, et_2=et_2)
average = b.Average(axis=1)(X=stack)
argmax = b.Argmax(axis=1)(X=average)
roc_auc = b.RocAuc()(gt_y=y, pred_probas=average)
pipeline = b.build(
{'X': X, 'y': y},
{'labels': argmax, 'probas': average, 'roc-auc': roc_auc}
)
GraphvizPainter(figure_dpi=100).from_pipeline(pipeline).render('pipeline.jpeg')
display(Image('pipeline.jpeg'))
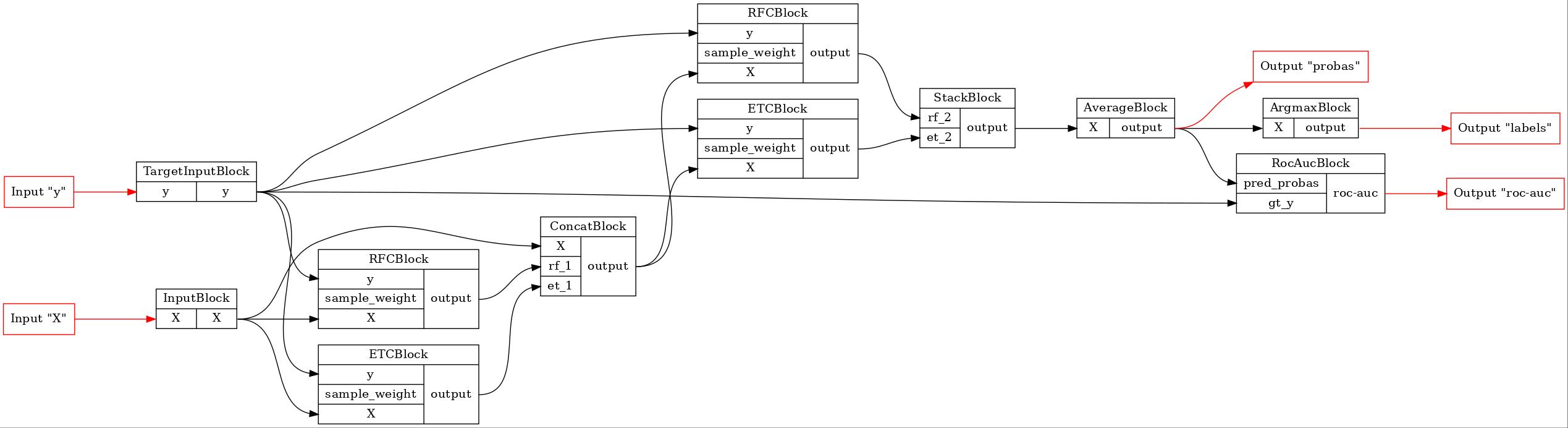
It’s a simple classification deep forest with 2 layers. Suppose we want to draw a confusion matrix for our pipeline. As we know, sklearn provides this functionality. So, let’s wrap our pipeline into the sklearn interface.
By default, BaseBoskPipelineWrapper
associates the X
, y
and sample_weigth
arguments of the fit method with the eponymous pipeline inputs. Output slots are expected to be named pred
and proba
. Thus, we need to specify mapping from the required pred
and proba
to our labels
and probas
outputs.
[3]:
sklearn_model = BoskPipelineClassifier(pipeline, outputs_map={'pred': 'labels', 'proba': 'probas'})
Let’s make some data, fit our classifier and draw the confusion matrix.
[4]:
iris = load_iris()
X = iris.data
y = iris.target
class_names = iris.target_names
X_train, X_test, y_train, y_test = train_test_split(X, y, random_state=42)
sklearn_model.fit(X_train, y_train)
ConfusionMatrixDisplay.from_estimator(
sklearn_model,
X_test,
y_test,
display_labels=class_names,
cmap='Greens',
normalize='true',
)
[4]:
<sklearn.metrics._plot.confusion_matrix.ConfusionMatrixDisplay at 0x7f62dae33d60>
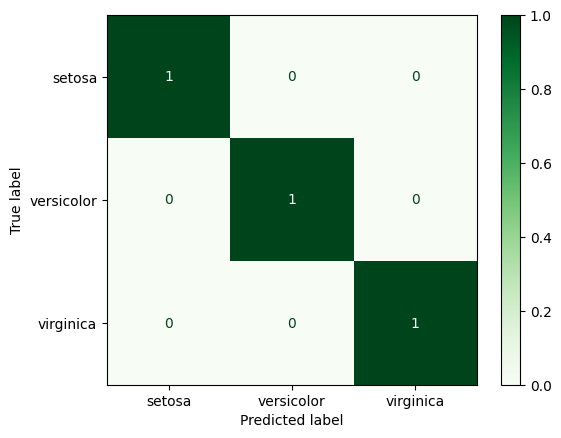